Data Readiness in the Age of Intelligence
AI is reshaping the enterprise—powering everything from smarter customer experiences to predictive operations. But powerful AI models and applications aren’t built on algorithms alone. They are built on data. If AI has become the latest engine of innovation, then data is the fuel. And the quality, condition, and formatting of that data has a significant impact on the performance of those models and applications. Amidst all the hype and excitement around AI, one foundational truth remains: models are only as good as the data they are trained on. It’s the age-old adage all over again: “Garbage in, garbage out.” But for those willing to do the work, the reverse can also be true.
So, what exactly is data readiness? It’s the process of transforming enterprise data from a chaotic liability into a strategic asset—clean, labeled, scalable, consolidated, and safe to use. Enterprises that want to unlock the full potential of AI must treat data readiness not as an afterthought, but as a core foundational component of their overall AI strategy. Here we will examine four different strategies that should be employed by organizations to improve their data readiness.
1. Data Refinement and Preprocessing
Most enterprise data is far from AI-ready. It lives in silos, arrives in inconsistent formats, and contains inaccuracies that would confuse even the most advanced algorithms. Preprocessing is the essential first step—where raw data is cleaned, reconciled, normalized, and transformed into something meaningful.
This is not glamorous work, but it is foundational. The best AI models in the world will fail if they’re trained on faulty data. Enterprises that prioritize preprocessing – those who treat data pipelines with the same rigor as software engineering – build the resilience and reliability that AI systems require. Done well, this transformation unlocks faster development cycles, better decision-making, and a clear line of sight into the business value of AI.
2. Data Labeling and Annotation
Artificial intelligence doesn’t emerge from data alone – it learns from examples. That’s where data annotation comes in. Labeling data with context – whether it’s classifying customer intent in emails, identifying patterns in transactions, or tagging product images – enables machines to understand what humans already know.
In the enterprise, annotation has moved beyond simple labels. It’s about encoding domain-specific expertise, capturing nuance, and doing so at scale. More importantly, it’s about doing it efficiently. Manual labeling remains essential for precision, but automation is accelerating the process. Enterprises are embracing systems that combine machine-generated annotations with human oversight, ensuring consistency without compromising speed.
3. Data Generation and Synthetic Data
Sometimes, the data you need simply doesn’t exist. It might be too rare to capture, too sensitive to share, or there just isn’t enough. Synthetic data offers a compelling answer: data that is generated – artificially, intelligently, and on demand. With the help of advanced models and simulations, enterprises are now generating realistic data to fill in the gaps. They’re training models on synthetic customer interactions, testing applications on simulated edge cases, and building prototypes before real-world data is even available.
4. Data Security and Protection
AI thrives on data – but so do security breaches, data leaks, and misuse. As enterprises unlock their data for AI, they must also safeguard it with equal intensity. Data readiness is not just about accessibility -- it’s also about protection. This means securing data across its lifecycle: controlling access, masking sensitive information, and ensuring that privacy and compliance are built into every workflow. As AI systems touch more personal and mission-critical data, the cost of getting it wrong grows exponentially.
In today’s regulatory landscape, data security is more than good practice -- it’s a competitive necessity. Customers, partners, and regulators are watching closely. Enterprises that lead with integrity – those who can say not only “our AI works” but also “our data is safe” – are the ones who will be best-positioned to win.
The Future Belongs to the Data-Ready
Data readiness is no longer a background task. It is foundational to any successful AI business strategy. It determines whether AI is a scalable engine of growth or a stalled experiment waiting for clean inputs. It is the difference between an operational success, and an R&D project that never leaves the lab. And the organizations that take it seriously are already separating themselves from the rest.
Being data-ready means more than managing infrastructure. It means curating and safeguarding your most valuable asset (your data) with precision, purpose, and vision. Because in the age of AI, success belongs to those who are ready – not just to deploy models, but to feed them with insight, structure, and trust.
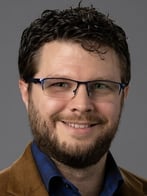
Justin “Hutch” Hutchens is an Innovation Principal at Trace3 and a leading voice in cybersecurity, risk management, and artificial intelligence. He is the author of “The Language of Deception: Weaponizing Next Generation AI,” a book focused on the adversarial risks of emerging AI technology. He is also a co-host of The Cyber Cognition Podcast, a show that explores the frontier of technological advancement and seeks to understand how cutting-edge technologies will transform our world. Hutch is a veteran of the United States Air Force, holds a Master’s degree in information systems, and routinely speaks at seminars, universities, and major global technology conferences.