5 Important Considerations when Starting the AI Agent Journey
Earlier this year, our Innovation Team published a comprehensive guide to deploying AI agents in the enterprise: AI Agents to the Rescue: Transforming Enterprise Automation. It outlined the huge potential of AI agents and how these work under the hood, including the different approaches and frameworks available today. And while the space is moving at a blistering pace with new solutions entering the market daily, there are some key considerations that will help you assess the maturity of internal processes and evaluate the overall readiness of your organization. So, as you gear up to lead the AI agent revolution, this blog can serve as a guide to the strategic decisions that need to be made today, to position your organization for success.
Why is This Time Different? Chatbot vs Copilot vs Agent
Even though the terms chatbot, copilot, and agent often get used interchangeably, big differences exist that explain AI agents’ recent emergence as the “holy grail” of automation. As the name suggests, the main differentiator is the degree of agency that these possess compared to tools in the past. Looking back, chatbots were mostly deterministic in nature, unable to tackle complex tasks or adapt to dynamic environments. While large language models fundamentally changed the type of interactions chatbots could facilitate on an enterprise level, LLM usage is also not without its challenges. LLMs mostly remain unreliable (“hallucinations”) and require extensive human supervision, explaining why many organizations are yet to realize true performance gains from their Gen AI initiatives. As a next evolution, Co-pilots were designed as GenAI-based interfaces to existing applications, offering users simplified ways to discover and augment existing features. However, copilots usually offer guidance without taking entire control from the user (e.g. meeting summaries or drafting emails). Agents on the other hand are designed to act autonomously with minimal supervision or intervention, adapting and executing goals in complex environments. While AI agents are powered by LLMs on the backend, agents can differ in the type of output they produce. AI agents are not limited to producing text, audio, or visual outputs, but they integrate with other enterprise tools to execute actions and achieve a certain goal or desired outcome. While this opens the door to new levels of task automation, this also comes with security and ethical considerations that will have to be tackled head-on.
Data Preparation & Process Maturity – Path to AI readiness
Many enterprises attempt to tackle AI without considering AI-specific data management issues, often leading to failed experiments or even failed deployments down the road. Following the “garbage-in-garbage-out” principle, AI agent performance is directly correlated with the quality and relevance of the data it is trained on. Ideally, the journey begins by defining and measuring minimum data standards for AI readiness early on for each use case. Good data management practices break down data silos and ensure efficient data access protocols and procedures, as well as consistently pre-processed, ready-to-use data. Other critical considerations often ignored in traditional data management practices include data bias, data labeling and drift. In some instances, additional datasets need to be acquired, licensed, or synthetically generated. Finally, data management activities don’t end once an agent has been developed. Deployment considerations and ongoing drift monitoring require dedicated data management practices.
Another important consideration is whether processes and workflows within an organization are mature enough for agentic AI. Depending on the use case and type of deployment, agents need to be grounded in a workflow-specific set of predefined actions or at least procedural knowledge, business context, and guardrails for each new process. This requires maturity and visibility into internal processes, collaborations, and tools used to execute tasks. Some of these will be more straightforward than others, as it can be difficult to understand the granular nuances of how a business process is really executed, especially when it's a human centric task. Solutions such as Skan.ai can provide real-time visibility into these processes and can promote AI readiness.
The Issue of Security – Autonomy vs Determinism
Unfortunately, AI agents not only unlock new levels of automation, they also create new attack vectors and business risks. This is because agents interact with different tools, external LLMs, or external agents to carry out tasks. These workflows and data paths create new attack surfaces such as APIs and uncontrolled data exchange that will have to be protected. And with the growing complexity of workflows taken on by agents, the governance of non-human identities (NHIs) will become even more challenging. Agents require access to applications, data, services etc. and often take on dynamically changing roles depending on the deployment context. Hence, unlike static service accounts, NHIs require management that’s far more nuanced – access management, handling credential delivery, permissions, and behavioral monitoring – all performed in real time.
Another risk factor is the level of autonomy that is given to an AI agent. Without a human in the loop, agents that are customer facing or that are dealing with highly sensitive data can cause significant harm. Given the probabilistic nature of the technology, it is crucial to balance agent autonomy with necessary human oversight. Especially in the beginning, agents will need temporary structures and guidance until they learn or develop own capabilities and become more proficient. Robust security measures, including automatic redaction and safe de-identification of sensitive data, audit logs, real-time monitoring, robust encryption of all data at rest and in transit, and explainability across all output results will ensure enterprise data remains private, secure, and compliant. As a prerequisite for deploying AI agents, organizations must establish clear process guardrails, including legal & ethical guidelines around autonomy and liability.
Use Case Selection
While AI agents have extremely wide applicability, it is important to identify specific processes and use cases that can benefit from agentic AI. This will help rationalize tool selection and resource allocation. More specifically, the complexity of the use case will explain the required level of sophistication of agentic systems. Single agent systems struggle with multi-step tasks that require navigating different contexts and managing dependencies. This means a single agent excels in more specific and narrow applications that are less sensitive. Examples are data analysis and reporting, generating creative elements, virtual assistants, and basic customer support. Multi-agent systems involve multiple specialized agents breaking down tasks and collaborating & coordinating their actions to achieve a certain objective. This allows them to tackle complex problems and workflows in changing environments, such as optimizing supply chains, mitigating risk, or automating customer service.
A good place to start your AI agent journey is by identifying basic roles that involve highly repetitive, deterministic tasks or workflows that can be automated more easily (e.g. document synthesis, RFPs etc.). From there, organizations can focus on horizontal solutions supporting customer service, IT, or sales workflows, or solutions that are more vertically focused, that automate workflows specific to the industry they operate in (e.g. patient care coordination in healthcare).
The Complex AI Agent Landscape Today
When evaluating different solutions, one of the key decisions to make is whether to build an agentic system in-house or buy off-the-shelf solutions. This is of course highly dependent on the use case at hand, but there are some general pros and cons for each option. Self-built agents can be customized for use cases across the organization and allow for the integration of advanced AI into existing systems, all while providing maximum control over sensitive data. However, this also requires technical talent and the stitching together of a variety of tools and frameworks to reach the desired functionality. Off-the shelf solutions on the other hand allow for quick time to value with pre-built templates and integrations. The downside here is that the technology might be limited to the most common use cases and any further customization or expansion of the platform may prove difficult. Hence, a trade-off exists between customization and control vs ease of deployment and cost.
It is also noteworthy how incumbent solutions are expanding into the world of agentic AI. For example, OpenAI’s recent launch of its o1 model made significant advances when it comes to reasoning, which is one of the key capabilities of AI agents. When given additional time to “think,” o1 can reason through a task holistically — planning ahead and performing a series of actions over an extended period of time that help the model arrive at an answer. This makes o1 well-suited for tasks that require synthesizing the results of multiple subtasks, like detecting privileged emails or brainstorming a product marketing strategy. This could position the company well to act as the foundation for any agentic system in the enterprise.
Below is a non-exhaustive overview of the emerging AI agent landscape today. It is important to note that many “traditional” AI vendors are trying to break into the world of AI agents and that capabilities vary greatly between different players.
Figure 1: Emerging AI Agent Landscape
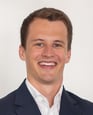
Lars is an Innovation Researcher on Trace3's Innovation Team, where he is focused on demystifying emerging trends & technologies across the enterprise IT space. By vetting innovative solutions, and combining insights from leading research and the world's most successful venture capital firms, Lars helps IT leaders navigate through an ever-changing technology landscape.