4 Words to Supercharge Your GenAI Security Strategy
Generative AI has unlocked numerous opportunities to enhance employee productivity. It spans areas like code generation, data analysis, knowledge management in tangible ways that fix code issues, create data visualizations, and find the answer in a host of documents. And these use cases are just scratching the surface of the benefits that it brings. In fact, use cases are so vast and the hype so high that it has caused an explosion of different applications leveraging GenAI. It’s to the point where organizations are feeling the pressure to adopt GenAI for staying ahead and maximizing productivity.
As organizations embrace GenAI for productivity gains, past lessons in security remain top of mind. This technology introduces new security attacks and concerns, such as prompt leak, prompt injection, inversion attacks, and reminds of the importance of good data security hygiene, like encryption. It also introduces a new form of shadow IT, where employees and teams might be so enthusiastic about the spread of GenAI solutions at their fingertips, that they start using it before it has been vetted through the organization. All these concerns are valid and are creating a market around securing GenAI throughout its lifecycle.
The question you might be wondering is – when should GenAI security become top of mind? And the answer is – as soon as you start considering enterprise-wide adoption or suspect it is being used within your organizations. Why so early? Because there a variety of different layers to consider navigating in this this attack surface landscape. Let’s dive in!
Ecosystem Dictates Security Approach
Organizations adopt GenAI in various ways, each tailored to their unique needs. This can include third-party GenAI applications like ChatGPT, tools that embed GenAI as a feature, such as GitHub Copilot, or proprietary GenAI solutions developed in-house. Each approach brings distinct advantages and considerations, enabling companies to integrate GenAI in ways that best align with their goals.
Because of how vast the use can be, it is important to first identify how GenAI is being used, both the known use and shadow AI. Then, start adopting security toolsets and best practices. This is because the types of use will determine which components are important to adopt. So, once you’ve identified your GenAI strategy, you’re ready for the next step. It’s time to introduce the 4 words that will build your security strategy.
Unpacking the Essential 4
Now, let’s unpack the four essential components of GenAI security: Govern, Shield, Test, and Protect. These categories create the pillars needed to secure GenAI from different angles. Let’s break them down one-by-one.
Govern
Goal: Ensure responsible and compliant use of GenAI by identifying, monitoring, and managing AI applications and identities within the organization.
Shadow AI
It is important to understand what your employees are accessing and what GenAI applications they may be exposed to. With this in mind, there are a variety of solutions that discover the different types of applications employees are accessing and determine how, and where, GenAI is being leveraged.
Sample Solutions: Nudge Security, Lasso Security, Acuvity
Lifecycle Governance
Across the GenAI security lifecycle, governance is important for ensuring overall ethical, legal, and operational procedures, regarding regulations and internal procedures. There are many pillars to GenAI lifecycle governance, and thus, solutions are specializing regulatory compliance, risk and control, and mitigation for specific threats.
Sample Solutions: Cranium, Onetrust, Dynamo AI, Credo AI, Acuvity
NHI
As GenAI use continues to adapt, so will the importance of expanding your controls. For example, there is evidence pointing to the potential of computers and agents working with applications more autonomously. As this becomes reality, non-human identity management will become increasingly important for establishing policies, compliance, and control mechanisms around the secure access of how AI NHI interact with applications and data.
Sample Solutions: Clutch Security, Astrix Security, Oasis Security
Shield
Goal: Implement protective barriers that intercept and filter AI interactions for maintaining data privacy and quality control in responses.
Prompt Firewall and Redaction
Understanding the data exchange between the application and model, solutions are creating firewalls that will intercept prompts and responses, redacting any necessary information. Organizations can configure these policies as needed to be helpful in situations like prompt attacks, PII and data loss prevention.
Sample Solutions: HiddenLayer, Lakera, Arthur, Prompt Security, Lasso Security, Island Security, LayerX, Acuvity, Dynamo AI
Quality Assurance
It is important not only to safeguard with a firewall, but also enable models to provide accurate information, regarding an organization's policies and brand alignment. From this perspective, quality assurance solutions are monitoring the model health across a variety of metrics, preventing hallucinations, blocking toxic and unapproved topics, ensuring everything is aligned with the organization.
Sample Solutions: Patronus, WhyLabs, Aporia
Test
Goal: Evaluate models to uncover and remediate vulnerabilities, ensuring security and reliability.
Model Testing
Testing models thoroughly creates the opportunity to swiftly identify and remediate security vulnerabilities within AI in a controlled environment. Solutions are spanning testing throughout development and deployment, including different test cases for uncovering risks. These tests include adversarial testing for prompt injection, prompt leaking, data leakage, as well as scanning for potential vulnerabilities within the models.
Sample Solutions: HiddenLayer, MindGard, Adversa, Advai, Patronus, Dynamo AI
Protect
Goal: Safeguard sensitive data throughout the GenAI lifecycle to maintain data integrity and compliance.
Confidential Computing
While data is being actively processed for inference, training and analysis, it becomes increasingly vulnerable of being exposed to a variety of different threats. By protecting data and applications running in secure enclaves, confidential computing prevents unauthorized access and manipulation, ensuring data privacy, regulatory compliance, and model integrity throughout the GenAI lifecycle.
Sample Solutions: Opaque, Fortanix
Encryption
As GenAI increasingly handles sensitive information, encryption ensures data remains protected. It keeps data secure, ensuring sensitive insights remain protected from unauthorized access and potential exposure. With various approaches, organizations can securely manage and control data throughout the lifecycle, enabling confident data sharing and analysis.
Sample Solutions: IroncoreLabs, Enveil
Training Data Protection
When training your models, there are two choices: use production data, or mock data. Since production data is not the safest option, solutions are facilitating the use of synthetic data in order to mimic real-world data patterns, detect bugs early and fix any privacy issues. This ensures your data is kept safe and model testing retains flexibility and compliance.
In summary
The truth is, while the GenAI hype is still new and emerging, AI itself has been around for a long time and securing it is still a work in progressing. Adding in generative to the AI conversations introduces whole new systems and classes of attacks. While we simplified this space into 4 key pillars, the evaluation process is complex. Organizations need to consider both current and future GenAI use cases, aligning each to the specific goals these pillars address. From there, it’s about selecting the right technologies to match these strategic objectives. Of course, as time goes on, it is expected that this market will continue to grow and change but it is important to start evaluating these technologies as soon as GenAI becomes a conversation in your organization.
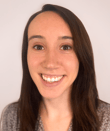
Kiersten Putnam is a Senior Innovation Researcher at Trace3. She is passionate about new innovative approaches that challenge traditional processes across the enterprise. As a member of the Innovation Team, she delivers research content on emerging trends and solutions across enterprise cloud, security, data, and infrastructure. When she's not researching, she is either exploring the surrounding areas of Denver, Colorado where she lives, or planning her next trip abroad.