Reframing Workplace Productivity: A new chapter in Knowledge Management and Enterprise Search
Organizations are constantly pursuing more efficient ways to search for information. As an organization scales, discovering and retrieving information becomes more and more challenging and productivity takes the hit. Innovations in this space include employing the use of deep learning models, knowledge graphs and now generative AI to connect content and context in an elegant manner that is promised to delight even the most decerning users. Emerging solutions integrate and index an organization’s most important data sources (incl. metadata). Considering content, people, and activity, solutions deliver a unified, personalized semantic search.
This blog post is a continuation from Trace3’s Innovation Team 2024 Enterprise Technology Themes series.
The Productivity Paradox of the Modern Workplace
Cloud computing has changed how most enterprises procure, deploy, and administer technology today. Gone are the days of physical infrastructure limitations and long complex licensing deals. Instead, modern IT teams are flexible in implementing new cloud-based solutions as business needs require. However, with organizations deploying more and more tools, they are increasingly left with SaaS sprawl and digital complexity.
At the same time, it has become easier than ever to produce unstructured information and share content, driven by recent developments in (Gen) AI. Everyday, knowledge is created (e.g. documents, messages, data points) that needs to be accessed across the organization to carry out daily tasks. However, when capturing knowledge, it is difficult to anticipate which departments and in what context it might be relied upon. This means knowledge workers spend a lot of time organizing information, creating folders etc. – while this structuring of knowledge might not be useful to others. Some knowledge might not even be formally documented, leading to loss of information over time without strong knowledge discovery capabilities.
While Enterprise Search platforms have been around for a long time, legacy tools have mostly underperformed. Most are difficult to connect to data sources and require manual search tuning, as well as painful tweaking of permissions to ensure security. These shortcomings are only made worse by the increasing complexity of the modern workplace.
Ultimately, this has resulted in employees spending a good chunk of their workday looking for information across tools (e.g. Slack, SharePoint, Salesforce), complicating collaboration across teams and negatively impacting employee experience, while increasing operational costs. Especially scenarios like onboarding or role changes that require lots of searching for information and experts are becoming gradually more challenging.
How Emerging Solutions are Reimagining Knowledge Retrieval
Given the highly dynamic work environments of today, it is no longer enough to collect and store information. A good knowledge management strategy now needs to consider how different teams & individuals find, use, and build on the valuable knowledge that an organization has painstakingly acquired over the years. To achieve this, solutions are combining several technologies into one holistic platform:
Data indexing - Modern platforms can crawl across a company’s different data sources and store both content and relevant metadata (e.g. file creator, date, update history etc.) within knowledge graphs. This is a big shift for search platforms, as knowledge graphs are able to connect data residing in disparate locations in a meaningful way, capturing not just the data itself but also relationships between data entities. However, directly querying a knowledge graph is not sufficiently performant to use for general search at enterprise scale. To unlock its benefits, knowledge graphs are used to generate contextual vector embeddings that can be indexed to make content easily searchable.
This is achieved by leveraging semantic vector search. Rather than just matching keywords, semantic search considers the context and relationships between search terms to establish user intent and identify the information required to respond to that intent. This means knowledge does not need to be perfectly organized beforehand – platforms can match every piece of information to the context and the situation in which it would be best used in. When searching the index and providing results back to the user, a solution should consider the organization’s unique language and structure, as well as usage patterns and of course the content itself. Hence, different users should receive different search results, depending on the specific context and without manual tuning.
Often times, content and data drawn from an initial localized semantic search can be passed on to Large Language Models (LLMs), such as OpenAI, in the form of a prompt. This means Generative AI workflows are grounded with context and recency to generate a response that synthesizes relevant and well-formed information for users – enabling additional productivity gains. Examples are code generation, text summarization, drafting customer support messages, etc. This process is referred to as Retrieval Augmented Generation (RAG).
Finally, across all these steps it is important to maintain tight security controls. A user should only be able to retrieve information that he/she has access to, in order to avoid data leakage across the company. As such, knowledge management platforms should integrate with existing IAM tools and be able to sync & enforce permissions from the underlying source system in real time.
Solutions
Overall, an effective knowledge management strategy that breaks down silos brings benefits that go beyond just time savings. Employees can collaborate in real time with quick access to experts and their work. Use cases range from supporting internal staff such as developers, sales teams or ITOps in their everyday work, to supporting external customers by enabling more self-service and quicker access to relevant information through chatbots or search tools. While some solutions are offering a holistic approach covering multiple use cases, other emerging solutions are taking a best-of-breed approach focusing on single use cases or taking a more internally vs externally focused approach.
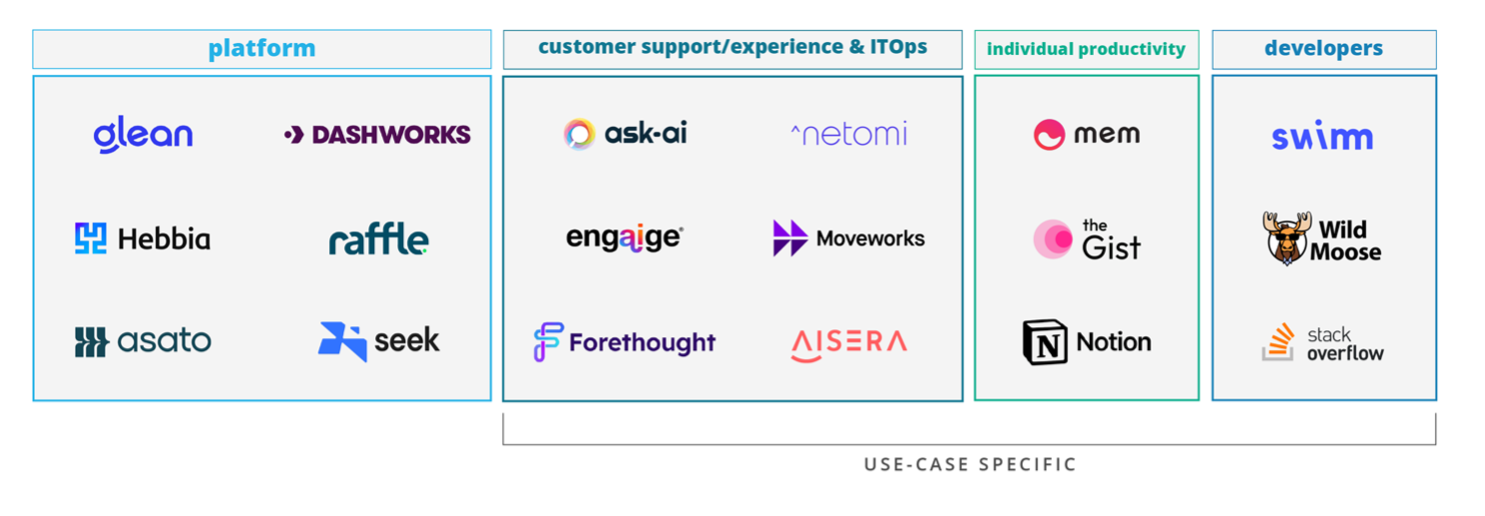
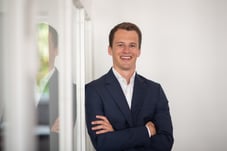